Search Engine Optimization Strategy Insights: Using Secondary Dimensions Efficiently
Wiki Article
Discover Deeper Insights With Second Measurements
Secondary dimensions serve as an effective device in the realm of information evaluation, providing a nuanced viewpoint that goes past surface-level observations. Keep tuned to uncover exactly how additional measurements can revolutionize the method you analyze and utilize your data for critical advantages.Advantages of Secondary Dimensions
One of the essential advantages of additional dimensions is the capacity to improve the context of the primary information. This included context allows experts to attract even more precise conclusions and make notified choices based on a much more extensive sight of the data. Secondary measurements help in giving a more holistic view of the relationships between various variables, consequently helping in the identification of underlying aspects that might influence the primary dataset.
Essentially, additional measurements play an essential function in enhancing data analysis procedures, using a much more nuanced perspective that can lead to useful understandings and workable recommendations.
Application Tips for Additional Dimensions
Carrying out secondary dimensions efficiently calls for a calculated technique that straightens with the specific purposes of the information analysis process. To begin, it is essential to clearly define the goals of the evaluation and identify the essential metrics that will offer the most valuable understandings - secondary dimensions. Select additional dimensions that complement the main dimensions and help in uncovering much deeper patterns or connections within the dataWhen executing secondary dimensions, it is important to think about the context in which the evaluation will certainly be carried out. Comprehending the audience and their info requirements will certainly assist the choice of pertinent secondary dimensions that add purposeful context to the key information points. Furthermore, make sure that the second dimensions selected work with the main measurements and can be efficiently compared or incorporated to extract useful understandings.
Moreover, it is recommended to check different mixes of additional and primary measurements to explore numerous point of views and uncover surprise connections within the data. Frequently refining the option and evaluating of second measurements based on the evolving logical demands will certainly ensure the analysis remains appropriate and informative.
Analyzing Information With Secondary Dimensions
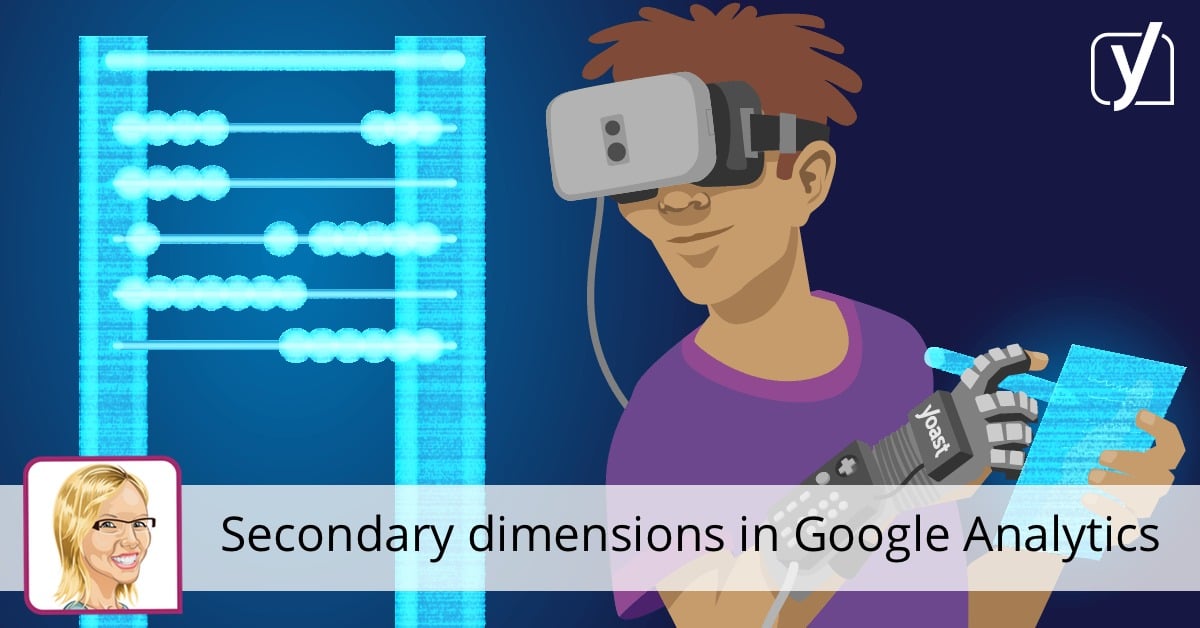
When assessing information with second measurements, it is vital to take into consideration how different variables communicate with one an additional. By cross-referencing key information with additional measurements, experts can discover relationships and dependences that provide a more holistic view of the data. This approach not only boosts the precision of understandings however likewise assists in making more informed decisions based upon her explanation the searchings for.
In addition, evaluating data with additional measurements allows the recognition of outliers or abnormalities that might influence the total analysis. By delving deeper right into the data through secondary dimensions, analysts can get a much more extensive understanding of the hidden variables driving the patterns observed in the key dataset.
Making The Most Of Insights Via Second Dimensions
To remove a greater level of deepness and precision from data analysis, leveraging second measurements is critical for taking full advantage of insights. By integrating secondary dimensions right into your evaluation, you can discover important relationships and patterns that may not be promptly obvious when taking a look at data through a main dimension alone. Additional measurements enable you to cut and dice your data better, offering a much more detailed understanding of the elements influencing your metrics.When utilized successfully, secondary measurements can boost the context visite site of your key data, using a more nuanced point of view on your analysis. As an example, by including second measurements such as time, location, or customer demographics, you can get a much deeper understanding of exactly how various sectors connect with your web content or items.
Moreover, secondary measurements can help you determine outliers, patterns, and correlations that could otherwise go undetected. By exploring your information from several angles, you can remove richer understandings and make more educated choices based on a comprehensive understanding of the hidden elements at play.
When Using Secondary Measurements,## Usual Mistakes to Prevent. secondary dimensions.
When integrating second measurements into data analysis, it is imperative to be mindful of typical errors that can prevent the removal of valuable insights. One common error is the abuse of second measurements without a clear purpose in mind. It is important to specify details goals and questions before selecting secondary measurements to ensure they align with the analysis purpose.
One more blunder to avoid is overcomplicating the analysis by including a lot of additional dimensions concurrently. This can cause details overload and make it testing to draw significant conclusions from the data. It is Extra resources advisable to start with a few relevant second measurements and gradually integrate much more as needed.
Additionally, ignoring data integrity issues can dramatically affect the precision of understandings originated from secondary dimensions. Unreliable or insufficient data can misshape the evaluation results and mislead decision-making processes. Routinely validating and cleaning the information is essential to ensure the reliability of the insights generated.
Verdict
In verdict, the critical application of additional dimensions in information evaluation uses a powerful tool for opening deeper understandings and boosting decision-making procedures. By incorporating added layers of details, analysts can acquire a much more comprehensive understanding of their dataset, uncover surprise fads, and determine essential elements affecting results. With careful factor to consider and application of additional dimensions, researchers can maximize the worth of their data and drive informed decision-making in numerous areas.Select second measurements that enhance the primary measurements and assist in discovering deeper patterns or correlations within the information.
Furthermore, ensure that the additional measurements selected are suitable with the main dimensions and can be successfully compared or integrated to extract important insights.
Using second measurements in data analysis improves the deepness and breadth of understandings acquired from the primary data points. By cross-referencing main information with second measurements, analysts can discover relationships and dependences that provide an even more all natural view of the data. By integrating second dimensions into your analysis, you can discover beneficial relationships and patterns that may not be quickly apparent when looking at data with a key measurement alone.
Report this wiki page